5 Revolutionary Techniques to Create Synthetic Data That Will Amaze You
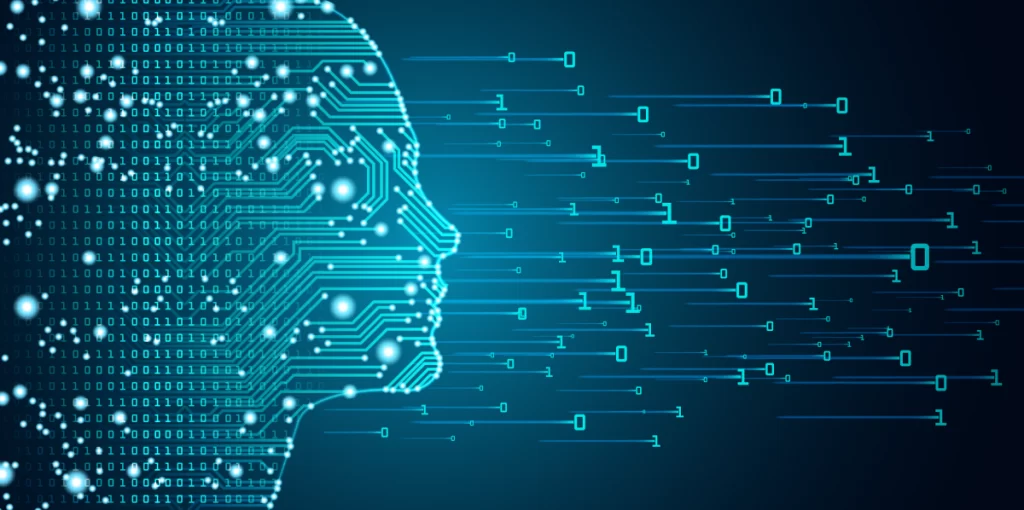
Synthetic data is a term that is becoming increasingly popular. It refers to artificially created
random data sets that can be used for various purposes, including data analysis, machine learning, and preserving privacy. This guide will provide an in-depth overview of five innovative and practical techniques for generating synthetic data, which have proven to be highly effective.
Table of Contents
Understanding Synthetic Data: A Brief Overview
Before diving into the techniques, let’s clarify what synthetic data is. Synthetic data is artificially generated data that mimics real-world data. It’s a valuable asset in scenarios where real data is scarce, sensitive, or difficult to obtain. By using synthetic data, organizations can ensure privacy, enhance data security, and improve the robustness of their data-driven models.Technique 1: Generative Adversarial Networks (GANs)
Generative Adversarial Networks, or GANs, are at the forefront of generating high-quality synthetic data. A GAN consists of two neural networks – the generator and the discriminator – that work in tandem. The generator creates data, while the discriminator evaluates it against real data. This competition drives the generator to produce increasingly realistic data, making GANs ideal for tasks like image and voice generation.Applications of GANs in Synthetic Data Creation
-
- Image and Video Generation: GANs can generate realistic images and videos, useful in fields like entertainment and virtual reality.
-
- Data Augmentation: They help in expanding datasets for training machine learning models, especially in cases where data is limited.
Technique 2: Data Augmentation
Data augmentation is a technique used to increase the diversity of your data set by making slight modifications to your existing data. This method is particularly useful in image and audio data sets.How Data Augmentation Benefits Synthetic Data Creation
-
- Enhancing Machine Learning Models: By providing varied data, models become more robust and less prone to overfitting.
-
- Balancing Datasets: It can help balance out datasets, especially in cases where some classes are underrepresented.
Technique 3: Simulation-Based Synthetic Data Generation
Simulation-based techniques involve creating a virtual environment where data is generated based on predefined rules and models. This method is particularly effective in scenarios where real-world data collection is impractical or impossible. An example of software that creates data based on modeling is Rndgen Data Generator.Key Advantages of Simulation-Based Techniques
-
- Controlled Environment: It allows for the generation of data under controlled conditions, ensuring consistency and reliability.
-
- Wide Range of Applications: From healthcare to aerospace, simulation-based synthetic data sets can be tailored to various industries.
Technique 4: Agent-Based Modeling
Agent-based modeling is a powerful technique where individual agents operate based on a set of rules. This method is used to generate data that reflects complex systems and interactions.The Power of Agent-Based Modeling in Data Generation
-
- Modeling Complex Systems: It’s ideal for scenarios like traffic systems, market dynamics, and social behavior analysis.
-
- Customizable: The rules can be tailored to fit the specific needs of the dataset required.
Technique 5: Deep Learning-Based Data Synthesis
Deep learning has revolutionized many aspects of technology, and synthetic data generation is no exception. By leveraging deep learning algorithms, synthetic data sets can be generated with high accuracy and realism.Deep Learning in Enhancing Synthetic Data Quality
-
- High-Quality Data: Deep learning models can generate data that closely mimic real-world scenarios.
-
- Versatility: These models can be applied to a wide range of data types, from text to complex 3D models.